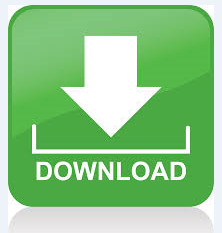
Regression analysis comes with several applications in finance. If the independent variables are highly correlated with each other, it will be difficult to assess the true relationships between the dependent and independent variables.
Non-collinearity: Independent variables should show a minimum correlation with each other.
However, since there are several independent variables in multiple linear analysis, there is another mandatory condition for the model: Multiple linear regression follows the same conditions as the simple linear model.
X 1, X 2, X 3 – Independent (explanatory) variables. The mathematical representation of multiple linear regression is: Y = a + b X 1 + c X 2 + d X 3 + ϵ Multiple linear regression analysis is essentially similar to the simple linear model, with the exception that multiple independent variables are used in the model.
Regression Analysis – Multiple Linear Regression The simple linear model is expressed using the following equation: Y = a + bX + ϵĬheck out the following video to learn more about simple linear regression: Simple linear regression is a model that assesses the relationship between a dependent variable and an independent variable. Regression Analysis – Simple Linear Regression
The residual (error) values follow the normal distribution. The value of the residual (error) is not correlated across all observations. The value of the residual (error) is constant across all observations. The value of the residual (error) is zero. The independent variable is not random. The dependent and independent variables show a linear relationship between the slope and the intercept. Linear regression analysis is based on six fundamental assumptions: Regression Analysis – Linear Model Assumptions Regression analysis offers numerous applications in various disciplines, including finance. Nonlinear regression analysis is commonly used for more complicated data sets in which the dependent and independent variables show a nonlinear relationship. The most common models are simple linear and multiple linear. Regression analysis includes several variations, such as linear, multiple linear, and nonlinear. It can be utilized to assess the strength of the relationship between variables and for modeling the future relationship between them. Regression analysis is a set of statistical methods used for the estimation of relationships between a dependent variable and one or more independent variables. Updated NovemWhat is Regression Analysis?